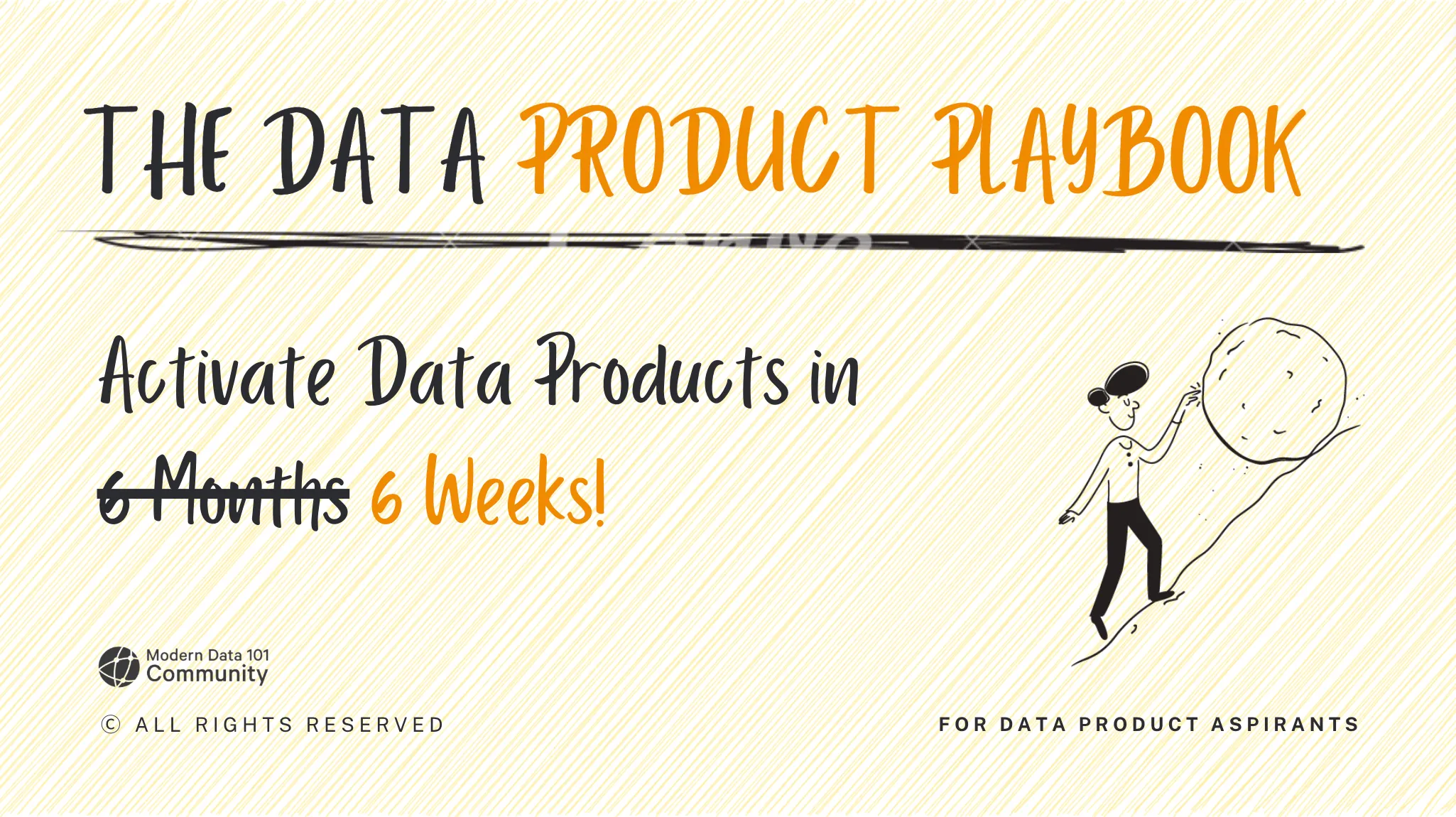
Here's your Playbook
Download now
Oops! Something went wrong while submitting the form.
Learn More
TABLE OF CONTENT
There's an idiom: “Like watching sausage getting made.” The idea being that you may like how sausage tastes, but that if you saw how sausage was made, you would find it a lot less appealing. The idiom applies not just to sausages but to the unsavory activities that are the backdrop for what we enjoy or admire, from law to medicine to politics to whatever.
~ The New Yorker
This holds true even in the realm of data, and “whatever” includes your very own data stack. We love the benefits Data Products earn for us but are averse to bringing product thinking into practice - not by choice but by years of habit imposed by traditional cycles.
But building a sausage machine is necessary to quickly produce sausages at scale and with a standard shape and consistency. These sausages are then delivered to masses in various formats, aka output ports, be it as hot dogs, pizza toppings, or sandwich filling - take your pick
The first thought that often strikes us is how vicious a munging and crunching machine could be. But in reality, when it comes to data, what mostly scares us is the black box effect. And anticipation that the data might cause digestive problems in downstream applications.
Be it sausage machinery or data products, it comes down to some fundamental product properties - ones we have unanimously agreed to be blind to for a very very long time in the data industry.
Such properties enable more transparency, confidence, and value from the processes and outcomes of the product.
The Differentiating Factor: Telling Products from Projects
The key difference between data products and data projects is how data interfaces with business metrics. As Don McGreal and Ralph Jockham point out in their extremely insightful book The Professional Product Owner:
How does it help the sausages? Intercepted with insights from McGreal & Jockham
Contrastingly, prevalent processes (aka “projects”) mean:
If there could be just one word to describe the nature of “projects”, it would be “fixed”. Projects are inanimate, defined and executed within the “scope” of a pre-defined success criteria. On the other hand, “products” are more “evolving”- living and breathing for the convenience of consumers. They are reactive by nature to the consumer’s changing needs. The criteria for “success” becomes a moving metric.
For simplicity’s sake, let’s see what we’d need to create these machines first, along with some examples of each instance.
From a product point of view, the above factors are not so different whenever ideating and executing development of most types of product which relatively delegate, dissipate, or redirect good amount of effort. For example, how a pen eliminates the need to dip into ink constantly, or how the chopping machine eliminates heavy labor through simple levers, and how bottles reduce roundtrips to water filters, and so on…
The production processes are ideally repeatable, governed, quality-assured, and resource-optimised. These are the fundamentals. No one would argue with these parameters laid out by a factory or workshop.
This shows us how the biggest barrier to data-as-a-product is, in fact, a mindset shift. We are so used to considering such parameters for regular products that, say, not aligning with business-driving metrics such as revenue or not regulating production end-to-end would just seem bizarre. But it doesn't seem bizarre for data, and ironically, the above fundamentals have often been perceived as bizarre when data products and data-as-a-product concepts started doing the rounds.
It holds true for the realm of data as well. And in this case, Data Products delegate, dissipate, or redirect good amount of cognitive effort. The production process is also repeatable, governed, quality-assured, and resource-optimised. And to create a Data Product, you need to focus on very similar instances behind product development.
Let’s visualise the same on a canvas, this time for data instead of 🍗
Too much work? Perhaps initially. And it’s always better than less returns on the dollars. Imagine if pen factories weren’t bothered about repeatability, quality, or speed/resource optimisation. Employees would be running around making one pen at a time for XYZ specifications from a random customer in one of the distribution shops. Isn’t it the same as spawning a whole new data pipeline to answer one specific business question or creating 1000K data warehouse tables being from 6K source tables?
Once the foundation, aka your Data Product Factory is ready, you could easily see the difference in speed, savings, reusability, and value for customers. That’s what would naturally happen with a product approach.
Want to deep-dive into repeatable production? Refer to Ch2 to Ch 5 in the End-to-End Data Product Guide.
Or, here’s a brief overview of the same with a complete view of building data products from its design to deployment, including its feedback loops: Data Product Lifecycle at a Glance